Research disemmination
Research disemmination#
Issue
Through the whole research cycle a range of outputs far beyond publications are produced, and each of them can have different levels of reproducibility and openness. For shared resources to be useful, they need to follow the FAIR principles [Wilkinson et al., 2016], to ensure they are: Findable (e.g., using persistent identifiers, such as Digital Object Identifiers (DOI) or Research Resource Identifiers (RRIDs), and described with rich metadata indexed in a searchable resource), Accessible (e.g., shared in public repositories, under open access or controlled access depending on regulations, so they can be retrievable by their identifier using standardized communication protocols), Interoperable (e.g., following a common standard for organization and vocabulary), and Reusable (e.g., richly described, with detailed provenance and an appropriate license). Indeed, without a license, materials (data, code, etc.) become unusable by the community due to the lack of permission and conditions for reuse, copy, modification, or distribution. Therefore, consenting through a license is essential for any material to be publicly shared.
See also
A useful general purpose resource, beyond neuroimaging, to find practical guidelines on reproducible research, project design, communication, collaboration, and ethics is The Turing Way (TTW, [The Turing Way Community et al., 2019], see the resources table). TTW is an open collaborative community-driven project, aiming to make data science accessible and comprehensible to ensure more reproducible and reusable projects.
What do we provide
TBE.
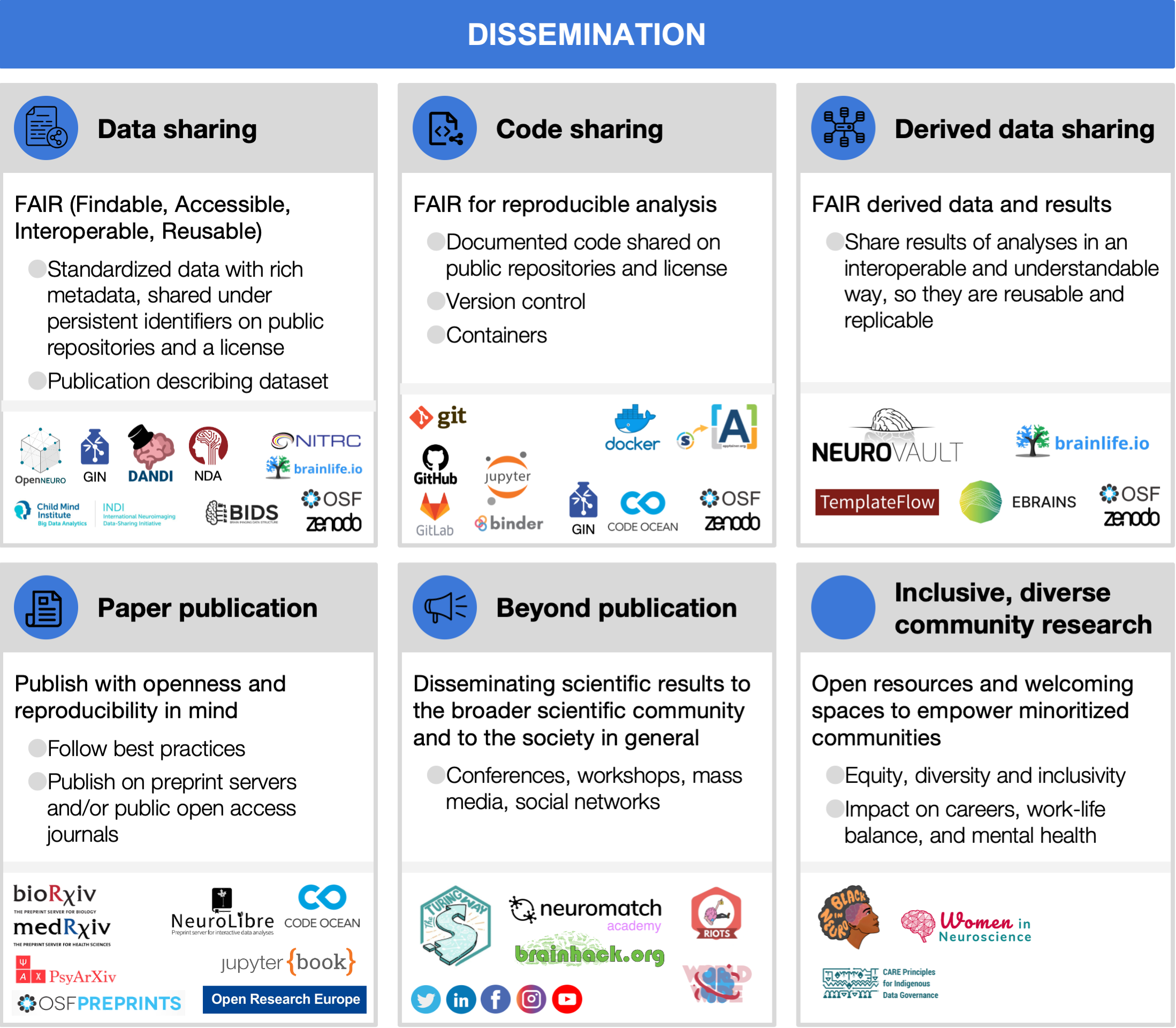
6.1 Data sharing
Making data available to the community is important for reproducibility, allows more scientific knowledge to be obtained from the same number of participants (animal or human), and also enables scientists to learn and teach others to reuse data, develop new analysis techniques, advance scientific hypotheses, and combine data in mega- or meta-analyses [Laird, 2021, Madan, 2021, Poldrack and Gorgolewski, 2014]. Moreover, the willingness to share has been shown to be positively related to the quality of the study [Aczel et al., 2021, Wicherts et al., 2011]. Because of the many advantages data sharing brings to the scientific community [Milham et al., 2018], more and more journals and funding agencies are requiring scientists to make their data public (curated and archived with a public record, but controlled access) or open (public data with uncontrolled access) upon the completion of the study, as long as it does not compromise participants’ privacy, legal regulations, or the ethical agreement between the researcher and participants (see Section 2.3 and Section 7).
For data to be interoperable and reusable, it should be organized following an accepted standard, such as BIDS (Section 4.1) and with at least a minimal set of metadata. Free data-sharing platforms are available for publicly sharing neuroimaging data, such as OpenNeuro [Markiewicz et al., 2021], brainlife [Avesani et al., 2019], GIN (G-Node Infrastructure), Distributed Archives for Neurophysiology Data Integration (DANDI), International Neuroimaging Data-Sharing Initiative (INDI), NeuroImaging Tools & Resources Collaborator (NITRC), etc. (see the resources table). Data could also be shared on institutional and funder archives such as the National Institute of Mental Health Data Archive (NDA); on dedicated repositories, such as the the Cambridge Centre for Ageing and Neuroscience, Cam-CAN [Shafto et al., 2014, Taylor et al., 2017] or The Open MEG Archive, OMEGA [Niso et al., 2016]; or on generic archives that are not neuroscience or neuroimaging specific, such as figshare, GitHub, the Open Science Framework, and Zenodo. If allowed by the law and participants’ consent (see Section 2.3), data sharing can be made open, or at least public.
Once curated and archived, data can further benefit the individual researcher, for example by adding them to the scientific literature in the form of data descriptors. Such an article type is not intended to communicate findings or interpretations but rather to provide detailed information about how the dataset was collected, what it includes and how it could be used, along with the shared data. In addition, an “open science badge” for data sharing is available in an increasing number of scientific journals [Kidwell et al., 2016] and some prizes are also available as a recognition for such efforts (e.g., OHBM’s Data Sharing prize).
It is important to note that there are unresolved issues with international data sharing that make it hard for some researchers to share their neuroimaging data. The two main issues are compliance with privacy regulations and credit. Privacy regulations can differ tremendously between cultural, legal, and ethical regions and these differences have an impact on whether certain data can be shared (e.g unprocessed MRI images) and if so, in which way (e.g. freely or after signing a data user agreement). Platforms that do not provide the necessary privacy protection mechanisms may not be an option for storing data from a jurisdiction that requires them. There is an ongoing discussion of the topic (see e.g [Eke et al., 2022, Jwa and Poldrack, 2022]) and solutions such as EBRAINs [Amunts et al., 2016, Amunts et al., 2019], a GDPR compatible data sharing platform in the Human Brain Project, as well as GDPR compatible consent forms (see Open Brain Consent, Section 2.3) are being developed. It can be expected that in the future data sharing procedures will undergo transformations as privacy laws will develop in more jurisdictions towards GDPR type laws (e.g. the Consumer Privacy Protection Act in Canada, or the California Consumer Privacy act). As for credit, sharing data with a DOI, or as a data paper when appropriate, allows the researchers to receive academic credit via citations.
6.2 Methodological transparency
Documenting performed analysis steps is key for reproducing studies’ results. For studies containing a small number of procedures, the methods section of an article could detail them in full length. This is, however, often not the case in current neuroimaging studies, where authors may need to summarize content in order to fit into the designated space, probably omitting relevant details. Therefore, the programming code itself becomes the most accurate source of the exact analysis steps performed on the data, and is anyway needed for reproducibility. Thus, it needs to be organized and clear (see recommendations suggested by [Sandve et al., 2013, van Vliet, 2020, Wilson et al., 2017], otherwise, results may not be reproducible, or even correct [Pavlov et al., 2021, Casadevall and Fang, 2014]. It should be noted that sharing an imperfect code is still much better than not sharing at all [Gorgolewski and Poldrack, 2016, Barnes, 2010].
While GUIs are a great and interactive way to learn to analyze data, one has to pay special attention to properly report the steps followed, as clicks are more difficult to be reported and reproduced than code [Pernet and Poline, 2015]. Some toolboxes using GUIs keep track of data ‘history’ and ‘provenance’ (e.g., Brainlife, Brainstorm, EEGLAB, FSL’s Feat tool) facilitating this task. Efforts are being put to improve these features.
To ensure long-term preservation of the shared code, we suggest using version control systems such as Git, and social coding platforms such as GitHub in combination with an archival database for assigning permanent DOI to code served for research, for instance, Zenodo [Troupin et al., 2018], brainlife.io/apps [Avesani et al., 2019] or Software Heritage [Di Cosmo, 2018]. These platforms help keep a snapshot of the version of the code used for the paper published, allowing exact reproduction in case of later code updates.
6.3 Derived data sharing
Sharing data derivatives is perhaps the most critical yet most challenging aspect of data sharing in support of reproducible science. The BIDS standard provides a general description of common derivative data (e.g., preprocessed data and statistical maps) and is actively working towards extending advanced specific derivatives for the different neuroimaging modalities. Yet as of today, standards for the description of advanced derivatives (such as activation or connectivity maps, or diffusion measures) are not available or mature for wide use. As a result, to date, the community lacks clear guidance and tools on how derived data should be organized to maximize its reuse and to encompass its provenance, and where such data could be shared.
Solutions for sharing derivatives comprise a mixture of in-house and semi-standardized data-tracking and representation methods. Examples of data-derivative sharing are the high-profile, centralized projects, such as the Human Connectome Projects [Van Essen et al., 2012], Ebrains of the Human Brain Project [Amunts et al., 2016, Amunts et al., 2019], the UK-Biobank [Alfaro-Almagro et al., 2016], the NKI-Rockland sample [Nooner et al., 2012], and the Adolescent Brain Cognitive Development (ABCD; [Feldstein Ewing and Luciana, 2018]) to name a few (see the resources table). These projects have developed project-specific solutions and in doing so also have provided a first-level implementation of what could be considered a data derivative standard. Yet, these projects are far from being open or community-developed as they must be centrally governed and mandated by the directives of the research plan.
As a result of the paucity of community-oriented standards, archives and software methods, sharing highly-processed neuroimaging data is still the frontier of reproducible science. One community-open archive for highly processed neuroimaging data derivatives is NeuroVault [Gorgolewski et al., 2015]. The archive accepts brain statistical maps derived from fMRI with the goal of being reused for meta-analytic studies. Data upload is open to researchers world-wide and the archive can accept brain maps submitted using most major formats but preferably using the NeuroImaging Data Model (NIDM; see Section 4.2). Another interesting example for automated and standardized composition and sharing of derived data is TemplateFlow [Ciric et al., 2021], which provides an open and distributed framework for establishment, access, management, and vetting of reference anatomies and atlases.
Another general neuroimaging platform, which allows lowering the barrier to sharing highly processed data derivatives, is Brainlife. Brainlife provides methods for publishing derivatives integrated with the data-processing applications used for generating results from the data via easy-to-use web interfaces for data-upload, processing, and publishing. Different licenses can be selected when publishing a record, allowing reuse of data and derivatives to other researchers (see sources that support the selection of an appropriate license listed in the resources table).
Sharing lighter-weight data products such as tables and figures is easier using generic repositories (e.g., OSF, Figshare, Github or Zenodo) under, for example, CC-BY license. This allows authors to retain rights on the figures they created, and others to re-use their figures freely, either in other publications or for educational purposes, while giving credit to the originating team. Additional material, such as slide presentations, or supporting content, should be shared using accessible formats (e.g., image files, pdf, PowerPoint slides, Markdown, jupyter notebooks, etc) via online repositories or institutional platforms, with appropriate licenses to indicate how the work could be reused. Whenever possible, using platforms that ensure long-term preservation is recommended (e.g., Zenodo, FigShare, OSF). Using platforms that provide a DOI is particularly encouraged, because it ensures that the shared data would be identifiable in the future.
6.4 Publication of scientific results
Scientific papers are currently the most important means for disseminating research results. However, they should also be written with reproducibility in mind. Guidelines to improve reproducibility can support the writing. The OHBM Committee on Best Practices in Data Analysis and Sharing (COBIDAS), has been promoting best practices, including open science. Recommendations from the committees for MRI {cite:p}{Nichols2017-qb} and MEG and EEG [Pernet et al., 2020] provide guidance on what to report. Other recent community efforts also led to guidelines for PET [Knudsen et al., 2020] and EEG reporting (e.g., Agreed Reporting Template for EEG Methodology - International Standard (ARTEM-IS) [Styles et al., 2021]). One tool that could help authors follow these guidelines while writing their report are their web-based apps (see the resources table). For the data description and preprocessing aspects, some tools (pyBIDS, bids-matlab) or pipelines (fMRIPrep) can also generate reports automatically, and/or method templates are provided (see Section 5.2). Exact description of methods is mandatory for reproducibility alongside detailed reporting of results.
In recent years, it has become very common in neuroimaging to publish papers as preprints, on servers such as bioRxiv, medRxiv, PsyArXiv or OSF (see the resources table), prior to peer review in scientific journals. Preprints are publicly available, expedite the process of releasing new findings, and also, importantly, allow authors to get feedback on their paper from a broader audience prior to final publication [Moshontz et al., 2021]. There are also initiatives for open community reviews of preprints, such as PREreview. Other initiatives have emerged to adapt to this paradigm shift, such as the recently launched Open Europe Research platform for publication and open peer review, which also includes the different outputs obtained throughout the research cycle (e.g., study protocol, data, methods and brief reports through the process) for research stemming from Horizon 2020 and Horizon Europe funding. In addition, novel publication formats have been developed, like NeuroLibre [Karakuzu et al., 2022], a preprint server to publish hybrid research objects including text, code, data, and runtime environment [DuPre et al., 2022]. More traditional publishers have successfully partnered with companies such as CodeOcean [Cheifet, 2021] to provide similar services.
Crucially, papers should be accessible to others, preferably to everyone. Many scientific publications are hidden behind paywalls, practically denying access from many people who could have gained from them. This is now slowly changing [Piwowar et al., 2018], with both researchers and funding agencies pushing towards open access, meaning that papers are fully open to all. Although the adoption of the concept of open access by major publishers is in itself a positive development, the way it was adopted could be considered arguable. For example, several journals considerably increased article processing charges [Budzinski et al., 2020, Khoo, 2019], increasingly excluding research produced in Low and Middle Income Countries from being published open access [Nabyonga-Orem et al., 2020]. Additionally, some publishers implement massive tracking technology with the argument to protect their rights and offer the data or derivatives of them for sale, as a recent report published by the German Research Foundation criticizes (DFG 2021). This raises many questions, related, for example, to the influence publishers and their algorithms will have in the future on strategic decisions of science institutions and freedom of science.
6.5 Beyond publication
The research lifecycle continues beyond paper publication. Disseminating scientific results to the broader scientific community and to the society in general is of utmost importance, to translate the newly acquired knowledge and give back to society. Oral and poster presentations at conferences contribute to the dissemination of results (including preliminary or intermediate results) within the scientific community, and also provide opportunities for feedback prior to publication. Workshops and other educational events contribute to expand the knowledge further and induce new communities. Popular and social media (e.g., press releases, interviews, podcasts, blog posts, twitter, facebook, linkedin, youtube, etc.) may reach an even wider and more heterogeneous audience. Different types of audiences may have different degrees of expertise and scientific knowledge, hence, for an effective communication, each of the outreach events should adapt accordingly (e.g., avoiding jargon and over interpretation, identifying your audience, promoting accessibility in content and language [Amano et al., 2021], and considering disabilities). See the TTW Guide for Communication for recommendations (https://the-turing-way.netlify.app/communication/communication.html). Slides presentations, and further outreach content should be shared FAIRly for higher impact (see Section 6.3).
Hackathons - such as the Brainhacks in the neuroimaging community [Gau et al., 2021] - typically offer times for “unconferences’’ in which attendees can propose a short talk to present some work-in-progress, an open question, or any other topic they wish to discuss with other participants. This deviates from more typical conferences in which only well-polished, finalized results can be presented. Other initiatives, such as Neuromatch Academy, Neurohackademy, OHBM Open Science Room, and Brainhack school MLT facilitate open science and provide opportunities for researchers to learn and get hands-on experience with open science practices, and also to engage with other researchers in the community. Those hackathons and the related online communities are also well-known as kick-starters for the development of community tools and standards in which researchers and engineers from different labs join forces. As those tools and standards get shaped, typically in multi-lab collaborations, researchers get the chance to exchange their views and practices. Overall, such events, slowly but surely, help shape a research culture that is driven by open collaborative communities rather than single groups of researchers.
6.6 Towards inclusive, diverse and community driven research
Taken to the next level, the described developments and introduced tools provide an opportunity for a paradigm shift: rather than carrying out a study from inception to results and only then disseminating the findings to the community, researchers now get multiple opportunities to share their ideas and results as they are being developed. Scientific research can now become more transparent, inclusive and collaborative throughout the research cycle.
In collaborative projects, having a well defined Code of Conduct is critical to ensure a welcoming and inclusive space for everyone regardless of background or identity. Initiatives such as TTW or the OHBM Conference have detailed Code of Conducts that can be of inspiration to adapt and use in new collaborative projects.
Community efforts should aim for equity, diversity and inclusivity. Unfortunately, as a reflection of our societies and cultures, the lack of these values is also prevalent in research and academia, contributing to gender bias, marginalization, and underrepresentation of racial, ethnic, and cultural minorities [Dworkin et al., 2020, Hofstra et al., 2020, Leslie et al., 2015]. These inequalities are produced at all stages, even at the time of recruiting participant samples for experiments [Forbes et al., 2021, Henrich et al., 2010, Laird, 2021] (see examples in the Section 2). Importantly, observing equity, diversity, and inclusivity in the recruitment of study participants can also improve the quality of research results (e.g., [Baggio et al., 2013]). Over the past years, the awareness and number of initiatives to mitigate bias and inequity at individual and institutional levels are growing [Levitis et al., 2021, Llorens et al., 2021, Malkinson et al., 2021, Schreiweis et al., 2019]. These aim to produce better research powered by a broader range of perspectives and ideas and to reduce the negative impact on the careers, work-life balance, and mental health of underrepresented groups. By providing open resources and promoting welcoming and inclusive spaces, we are also improving access to the tools, training and infrastructure which can facilitate reproducible research, which will accelerate discoveries, and ultimately, advance science.
References on this page
- F1
Balazs Aczel, Barnabas Szaszi, Gustav Nilsonne, Olmo R van den Akker, Casper J Albers, Marcel Alm van Assen, Jojanneke A Bastiaansen, Daniel Benjamin, Udo Boehm, Rotem Botvinik-Nezer, Laura F Bringmann, Niko A Busch, Emmanuel Caruyer, Andrea M Cataldo, Nelson Cowan, Andrew Delios, Noah Nn van Dongen, Chris Donkin, Johnny B van Doorn, Anna Dreber, Gilles Dutilh, Gary F Egan, Morton Ann Gernsbacher, Rink Hoekstra, Sabine Hoffmann, Felix Holzmeister, Juergen Huber, Magnus Johannesson, Kai J Jonas, Alexander T Kindel, Michael Kirchler, Yoram K Kunkels, D Stephen Lindsay, Jean-Francois Mangin, Dora Matzke, Marcus R Munafò, Ben R Newell, Brian A Nosek, Russell A Poldrack, Don van Ravenzwaaij, Jörg Rieskamp, Matthew J Salganik, Alexandra Sarafoglou, Tom Schonberg, Martin Schweinsberg, David Shanks, Raphael Silberzahn, Daniel J Simons, Barbara A Spellman, Samuel St-Jean, Jeffrey J Starns, Eric Luis Uhlmann, Jelte Wicherts, and Eric-Jan Wagenmakers. Consensus-based guidance for conducting and reporting multi-analyst studies. Elife, November 2021.
- F2
missing booktitle in Alfaro-Almagro2016-pj
- F3
Tatsuya Amano, Clarissa Rios Rojas, Yap Boum, Ii, Margarita Calvo, and Biswapriya B Misra. Ten tips for overcoming language barriers in science. Nature Human Behaviour, 5(9):1119–1122, September 2021.
- F4(1,2)
Katrin Amunts, Christoph Ebell, Jeff Muller, Martin Telefont, Alois Knoll, and Thomas Lippert. The human brain project: creating a european research infrastructure to decode the human brain. Neuron, 92(3):574–581, November 2016.
- F5(1,2)
Katrin Amunts, Alois C Knoll, Thomas Lippert, Cyriel M A Pennartz, Philippe Ryvlin, Alain Destexhe, Viktor K Jirsa, Egidio D'Angelo, and Jan G Bjaalie. The human brain project: synergy between neuroscience, computing, informatics, and brain-inspired technologies. PLoS Biology, 17(7):e3000344, July 2019.
- F6(1,2)
Paolo Avesani, Brent McPherson, Soichi Hayashi, Cesar F Caiafa, Robert Henschel, Eleftherios Garyfallidis, Lindsey Kitchell, Daniel Bullock, Andrew Patterson, Emanuele Olivetti, Olaf Sporns, Andrew J Saykin, Lei Wang, Ivo Dinov, David Hancock, Bradley Caron, Yiming Qian, and Franco Pestilli. The open diffusion data derivatives, brain data upcycling via integrated publishing of derivatives and reproducible open cloud services. Scientific Data, 6(1):69, May 2019.
- F7
Giovannella Baggio, Alberto Corsini, Annarosa Floreani, Sandro Giannini, and Vittorina Zagonel. Gender medicine: a task for the third millennium. Clinical Chemistry and Laboratory Medicine, 51(4):713–727, April 2013.
- F8
Nick Barnes. Publish your computer code: it is good enough. Nature, 467(7317):753, October 2010.
- F9
Oliver Budzinski, Thomas Grebel, Jens Wolling, and Xijie Zhang. Drivers of article processing charges in open access. SSRN Electronic Journal, 124:2185–2206, 2020.
- F10
Arturo Casadevall and Ferric C Fang. Causes for the persistence of impact factor mania. MBio, 5(2):e00064–14, March 2014.
- F11
Barbara Cheifet. Promoting reproducibility with code ocean. Genome Biology, 22(1):65, February 2021.
- F12
missing note in Ciric2021-uw
- F13
missing booktitle in Di_Cosmo2018-rb
- F14
Elizabeth DuPre, Chris Holdgraf, Agah Karakuzu, Loïc Tetrel, Pierre Bellec, Nikola Stikov, and Jean-Baptiste Poline. Beyond advertising: new infrastructures for publishing integrated research objects. PLoS Computational Biology, 18(1):e1009651, January 2022.
- F15
Jordan D Dworkin, Kristin A Linn, Erin G Teich, Perry Zurn, Russell T Shinohara, and Danielle S Bassett. The extent and drivers of gender imbalance in neuroscience reference lists. Nat. Neurosci., 23(8):918–926, August 2020.
- F16
Damian Eke, Amy Bernard, Jan G Bjaalie, Ricardo Chavarriaga, Takashi Hanakawa, Anthony Hannan, Sean Hill, Maryann Elizabeth Martone, Agnes McMahon, Oliver Ruebel, Edda Thiels, and Franco Pestilli. International data governance for neuroscience. Neuron, 110(4):600–612, 2022.
- F17
S Feldstein Ewing and M Luciana. The adolescent brain cognitive development (ABCD) consortium: rationale, aims, and assessment strategy [special issue]. Developmental Cognitive Neuroscience, 32:1–164, 2018.
- F18
Samuel H Forbes, Prerna Aneja, and Olivia Guest. The myth of (a)typical development. PsyArXiv, October 2021.
- F19
Rémi Gau, Stephanie Noble, Katja Heuer, Katherine L Bottenhorn, Isil P Bilgin, Yu-Fang Yang, Julia M Huntenburg, Johanna M M Bayer, Richard A I Bethlehem, Shawn A Rhoads, Christoph Vogelbacher, Valentina Borghesani, Elizabeth Levitis, Hao-Ting Wang, Sofie Van Den Bossche, Xenia Kobeleva, Jon Haitz Legarreta, Samuel Guay, Selim Melvin Atay, Gael P Varoquaux, Dorien C Huijser, Malin S Sandström, Peer Herholz, Samuel A Nastase, Amanpreet Badhwar, Guillaume Dumas, Simon Schwab, Stefano Moia, Michael Dayan, Yasmine Bassil, Paula P Brooks, Matteo Mancini, James M Shine, David O'Connor, Xihe Xie, Davide Poggiali, Patrick Friedrich, Anibal S Heinsfeld, Lydia Riedl, Roberto Toro, César Caballero-Gaudes, Anders Eklund, Kelly G Garner, Christopher R Nolan, Damion V Demeter, Fernando A Barrios, Junaid S Merchant, Elizabeth A McDevitt, Robert Oostenveld, R Cameron Craddock, Ariel Rokem, Andrew Doyle, Satrajit S Ghosh, Aki Nikolaidis, Olivia W Stanley, Eneko Uruñuela, and Brainhack Community. Brainhack: developing a culture of open, inclusive, community-driven neuroscience. Neuron, 109(11):1769–1775, June 2021.
- F20
Krzysztof J Gorgolewski and Russell A Poldrack. A practical guide for improving transparency and reproducibility in neuroimaging research. PLoS Biology, 14(7):e1002506, July 2016.
- F21
Krzysztof J Gorgolewski, Gael Varoquaux, Gabriel Rivera, Yannick Schwarz, Satrajit S Ghosh, Camille Maumet, Vanessa V Sochat, Thomas E Nichols, Russell A Poldrack, Jean-Baptiste Poline, Tal Yarkoni, and Daniel S Margulies. NeuroVault.org: a web-based repository for collecting and sharing unthresholded statistical maps of the human brain. Frontiers in Neuroinformatics, 9:8, April 2015.
- F22
Joseph Henrich, Steven J Heine, and Ara Norenzayan. The weirdest people in the world? Behavioral and Brain Sciences, 33(2-3):61–83, June 2010.
- F23
B Hofstra, V V Kulkarni, Galvez S Munoz-Najar, B He, D Jurafsky, and D A McFarland. The diversity–innovation paradox in science. Proceedings of the National Academy of Sciences (PNAS), 117(17):9284–9291, 2020.
- F24
Anita S Jwa and Russell A Poldrack. The spectrum of data sharing policies in neuroimaging data repositories. Hum. Brain Mapp., 43(8):2707–2721, June 2022.
- F25
missing journal in Karakuzu:2022wq
- F26
Shaun Yon-Seng Khoo. Article processing charge hyperinflation and price insensitivity: an open access sequel to the serials crisis. 2019.
- F27
Mallory C Kidwell, Ljiljana B Lazarević, Erica Baranski, Tom E Hardwicke, Sarah Piechowski, Lina-Sophia Falkenberg, Curtis Kennett, Agnieszka Slowik, Carina Sonnleitner, Chelsey Hess-Holden, Timothy M Errington, Susann Fiedler, and Brian A Nosek. Badges to acknowledge open practices: a simple, low-cost, effective method for increasing transparency. PLoS Biology, 14(5):e1002456, May 2016.
- F28
Gitte M Knudsen, Melanie Ganz, Stefan Appelhoff, Ronald Boellaard, Guy Bormans, Richard E Carson, Ciprian Catana, Doris Doudet, Antony D Gee, Douglas N Greve, Roger N Gunn, Christer Halldin, Peter Herscovitch, Henry Huang, Sune H Keller, Adriaan A Lammertsma, Rupert Lanzenberger, Jeih-San Liow, Talakad G Lohith, Mark Lubberink, Chul H Lyoo, J John Mann, Granville J Matheson, Thomas E Nichols, Martin Nørgaard, Todd Ogden, Ramin Parsey, Victor W Pike, Julie Price, Gaia Rizzo, Pedro Rosa-Neto, Martin Schain, Peter Jh Scott, Graham Searle, Mark Slifstein, Tetsuya Suhara, Peter S Talbot, Adam Thomas, Mattia Veronese, Dean F Wong, Maqsood Yaqub, Francesca Zanderigo, Sami Zoghbi, and Robert B Innis. Guidelines for the content and format of PET brain data in publications and archives: a consensus paper. Journal of Cerebral Blood Flow and Metabolism, 40(8):1576–1585, August 2020.
- F29(1,2)
Angela R Laird. Large, open datasets for human connectomics research: considerations for reproducible and responsible data use. Neuroimage, 244:118579, December 2021.
- F30
Sarah-Jane Leslie, Andrei Cimpian, Meredith Meyer, and Edward Freeland. Expectations of brilliance underlie gender distributions across academic disciplines. Science, 347(6219):262–265, January 2015.
- F31
Elizabeth Levitis, Cassandra D Gould van Praag, Rémi Gau, Stephan Heunis, Elizabeth DuPre, Gregory Kiar, Katherine L Bottenhorn, Tristan Glatard, Aki Nikolaidis, Kirstie Jane Whitaker, Matteo Mancini, Guiomar Niso, Soroosh Afyouni, Eva Alonso-Ortiz, Stefan Appelhoff, Aurina Arnatkeviciute, Selim Melvin Atay, Tibor Auer, Giulia Baracchini, Johanna M M Bayer, Michael J S Beauvais, Janine D Bijsterbosch, Isil P Bilgin, Saskia Bollmann, Steffen Bollmann, Rotem Botvinik-Nezer, Molly G Bright, Vince D Calhoun, Xiao Chen, Sidhant Chopra, Hu Chuan-Peng, Thomas G Close, Savannah L Cookson, R Cameron Craddock, Alejandro De La Vega, Benjamin De Leener, Damion V Demeter, Paola Di Maio, Erin W Dickie, Simon B Eickhoff, Oscar Esteban, Karolina Finc, Matteo Frigo, Saampras Ganesan, Melanie Ganz, Kelly G Garner, Eduardo A Garza-Villarreal, Gabriel Gonzalez-Escamilla, Rohit Goswami, John D Griffiths, Tijl Grootswagers, Samuel Guay, Olivia Guest, Daniel A Handwerker, Peer Herholz, Katja Heuer, Dorien C Huijser, Vittorio Iacovella, Michael J E Joseph, Agah Karakuzu, David B Keator, Xenia Kobeleva, Manoj Kumar, Angela R Laird, Linda J Larson-Prior, Alexandra Lautarescu, Alberto Lazari, Jon Haitz Legarreta, Xue-Ying Li, Jinglei Lv, Sina Mansour L, David Meunier, Dustin Moraczewski, Tulika Nandi, Samuel A Nastase, Matthias Nau, Stephanie Noble, Martin Norgaard, Johnes Obungoloch, Robert Oostenveld, Edwina R Orchard, Ana Luísa Pinho, Russell A Poldrack, Anqi Qiu, Pradeep Reddy Raamana, Ariel Rokem, Saige Rutherford, Malvika Sharan, Thomas B Shaw, Warda T Syeda, Meghan M Testerman, Roberto Toro, Sofie L Valk, Sofie Van Den Bossche, Gaël Varoquaux, František Váša, Michele Veldsman, Jakub Vohryzek, Adina S Wagner, Reubs J Walsh, Tonya White, Fu-Te Wong, Xihe Xie, Chao-Gan Yan, Yu-Fang Yang, Yohan Yee, Gaston E Zanitti, Ana E Van Gulick, Eugene Duff, and Camille Maumet. Centering inclusivity in the design of online conferences: an OHBM-Open science perspective. GigaScience, August 2021.
- F32
Anaïs Llorens, Athina Tzovara, Ludovic Bellier, Ilina Bhaya-Grossman, Aurélie Bidet-Caulet, William K Chang, Zachariah R Cross, Rosa Dominguez-Faus, Adeen Flinker, Yvonne Fonken, Mark A Gorenstein, Chris Holdgraf, Colin W Hoy, Maria V Ivanova, Richard T Jimenez, Soyeon Jun, Julia W Y Kam, Celeste Kidd, Enitan Marcelle, Deborah Marciano, Stephanie Martin, Nicholas E Myers, Karita Ojala, Anat Perry, Pedro Pinheiro-Chagas, Stephanie K Riès, Ignacio Saez, Ivan Skelin, Katarina Slama, Brooke Staveland, Danielle S Bassett, Elizabeth A Buffalo, Adrienne L Fairhall, Nancy J Kopell, Laura J Kray, Jack J Lin, Anna C Nobre, Dylan Riley, Anne-Kristin Solbakk, Joni D Wallis, Xiao-Jing Wang, Shlomit Yuval-Greenberg, Sabine Kastner, Robert T Knight, and Nina F Dronkers. Gender bias in academia: a lifetime problem that needs solutions. Neuron, 109(13):2047–2074, July 2021.
- F33
Christopher R Madan. Scan once, analyse many: using large Open-Access neuroimaging datasets to understand the brain. Neuroinformatics, May 2021.
- F34
missing note in Malkinson2021-tg
- F35
Christopher J Markiewicz, Krzysztof J Gorgolewski, Franklin Feingold, Ross Blair, Yaroslav O Halchenko, Eric Miller, Nell Hardcastle, Joe Wexler, Oscar Esteban, Mathias Goncavles, Anita Jwa, and Russell Poldrack. The OpenNeuro resource for sharing of neuroscience data. Elife, October 2021.
- F36
Michael P Milham, R Cameron Craddock, Jake J Son, Michael Fleischmann, Jon Clucas, Helen Xu, Bonhwang Koo, Anirudh Krishnakumar, Bharat B Biswal, F Xavier Castellanos, Stan Colcombe, Adriana Di Martino, Xi-Nian Zuo, and Arno Klein. Assessment of the impact of shared brain imaging data on the scientific literature. Nat. Commun., 9(1):2818, July 2018.
- F37
Hannah Moshontz, Grace Binion, Haley Walton, Benjamin T Brown, and Moin Syed. A guide to posting and managing preprints. Advances in Methods and Practices in Psychological Science, April 2021.
- F38
Juliet Nabyonga-Orem, James Avoka Asamani, Thomas Nyirenda, and Seye Abimbola. Article processing charges are stalling the progress of african researchers: a call for urgent reforms. BMJ Glob Health, September 2020.
- F39
Guiomar Niso, Christine Rogers, Jeremy T Moreau, Li-Yuan Chen, Cecile Madjar, Samir Das, Elizabeth Bock, François Tadel, Alan C Evans, Pierre Jolicoeur, and Sylvain Baillet. OMEGA: the open MEG archive. Neuroimage, 124(Pt B):1182–1187, January 2016.
- F40
Kate Brody Nooner, Stanley Colcombe, Russell Tobe, Maarten Mennes, Melissa Benedict, Alexis Moreno, Laura Panek, Shaquanna Brown, Stephen Zavitz, Qingyang Li, Sharad Sikka, David Gutman, Saroja Bangaru, Rochelle Tziona Schlachter, Stephanie Kamiel, Ayesha Anwar, Caitlin Hinz, Michelle Kaplan, Anna Rachlin, Samantha Adelsberg, Brian Cheung, Ranjit Khanuja, Chaogan Yan, Cameron Craddock, Vincent Calhoun, William Courtney, Margaret King, Dylan Wood, Christine Cox, Clare Kelly, Adriana DiMartino, Eva Petkova, Philip Reiss, Nancy Duan, Dawn Thompsen, Bharat Biswal, Barbara Coffey, Matthew Hoptman, Daniel C Javitt, Nunzio Pomara, John Sidtis, Harold Koplewicz, Francisco Xavier Castellanos, Bennett Leventhal, and Michael Milham. The NKI-Rockland sample: a model for accelerating the pace of discovery science in psychiatry. Frontiers in Neuroscience, 2012.
- F41
Yuri G Pavlov, Nika Adamian, Stefan Appelhoff, Mahnaz Arvaneh, Christopher S Y Benwell, Christian Beste, Amy R Bland, Daniel E Bradford, Florian Bublatzky, Niko A Busch, Peter E Clayson, Damian Cruse, Artur Czeszumski, Anna Dreber, Guillaume Dumas, Benedikt Ehinger, Giorgio Ganis, Xun He, José A Hinojosa, Christoph Huber-Huber, Michael Inzlicht, Bradley N Jack, Magnus Johannesson, Rhiannon Jones, Evgenii Kalenkovich, Laura Kaltwasser, Hamid Karimi-Rouzbahani, Andreas Keil, Peter König, Layla Kouara, Louisa Kulke, Cecile D Ladouceur, Nicolas Langer, Heinrich R Liesefeld, David Luque, Annmarie MacNamara, Liad Mudrik, Muthuraman Muthuraman, Lauren B Neal, Gustav Nilsonne, Guiomar Niso, Sebastian Ocklenburg, Robert Oostenveld, Cyril R Pernet, Gilles Pourtois, Manuela Ruzzoli, Sarah M Sass, Alexandre Schaefer, Magdalena Senderecka, Joel S Snyder, Christian K Tamnes, Emmanuelle Tognoli, Marieke K van Vugt, Edelyn Verona, Robin Vloeberghs, Dominik Welke, Jan R Wessel, Ilya Zakharov, and Faisal Mushtaq. #EEGManyLabs: investigating the replicability of influential EEG experiments. Cortex, 144:213–229, April 2021.
- F42
Cyril R Pernet, Ramon Martinez-Cancino, Dung Truong, Scott Makeig, and Arnaud Delorme. From BIDS-Formatted EEG data to Sensor-Space group results: a fully reproducible workflow with EEGLAB and LIMO EEG. Front. Neurosci., 14:610388, 2020.
- F43
Cyril R Pernet and Jean-Baptiste Poline. Improving functional magnetic resonance imaging reproducibility. GigaScience, 2015.
- F44
Heather A Piwowar, Jason Priem, Vincent Larivière, Juan Pablo Alperin, Lisa Matthias, Bree Norlander, Ashley Farley, Jevin West, and Stefanie Haustein. The state of OA: a large-scale analysis of the prevalence and impact of open access articles. PeerJ, 6:e4375, February 2018.
- F45
Russell A Poldrack and Krzysztof J Gorgolewski. Making big data open: data sharing in neuroimaging. Nature Neuroscience, 17(11):1510–1517, November 2014.
- F46
Geir Kjetil Sandve, Anton Nekrutenko, James Taylor, and Eivind Hovig. Ten simple rules for reproducible computational research. PLoS Computational Biology, 9(10):e1003285, October 2013.
- F47
Christiane Schreiweis, Emmanuelle Volle, Alexandra Durr, Alexandra Auffret, Cécile Delarasse, Nathalie George, Magali Dumont, Bassem A Hassan, Nicolas Renier, Charlotte Rosso, Michel Thiebaut de Schotten, Eric Burguière, and Violetta Zujovic. A neuroscientific approach to increase gender equality. Nature Human Behaviour, 3(12):1238–1239, September 2019.
- F48
Meredith A Shafto, Cam-CAN, Lorraine K Tyler, Marie Dixon, Jason R Taylor, James B Rowe, Rhodri Cusack, Andrew J Calder, William D Marslen-Wilson, John Duncan, Tim Dalgleish, Richard N Henson, Carol Brayne, and Fiona E Matthews. The cambridge centre for ageing and neuroscience (Cam-CAN) study protocol: a cross-sectional, lifespan, multidisciplinary examination of healthy cognitive ageing. 2014.
- F49
Suzy J Styles, Vanja Ković, Han Ke, and Anđela Šoškić. Towards ARTEM-IS: design guidelines for evidence-based EEG methodology reporting tools. Neuroimage, 245:118721, December 2021.
- F50
Jason R Taylor, Nitin Williams, Rhodri Cusack, Tibor Auer, Meredith A Shafto, Marie Dixon, Lorraine K Tyler, Cam-Can, and Richard N Henson. The cambridge centre for ageing and neuroscience (Cam-CAN) data repository: structural and functional MRI, MEG, and cognitive data from a cross-sectional adult lifespan sample. Neuroimage, 144(Pt B):262–269, January 2017.
- F51
missing booktitle in Troupin2018-px
- F52
D C Van Essen, K Ugurbil, E Auerbach, D Barch, T E J Behrens, R Bucholz, A Chang, L Chen, M Corbetta, S W Curtiss, S Della Penna, D Feinberg, M F Glasser, N Harel, A C Heath, L Larson-Prior, D Marcus, G Michalareas, S Moeller, R Oostenveld, S E Petersen, F Prior, B L Schlaggar, S M Smith, A Z Snyder, J Xu, E Yacoub, and WU-Minn HCP Consortium. The human connectome project: a data acquisition perspective. Neuroimage, 62(4):2222–2231, October 2012.
- F53
Marijn van Vliet. Seven quick tips for analysis scripts in neuroimaging. PLoS Computational Biology, 16(3):e1007358, March 2020.
- F54
Jelte M Wicherts, Marjan Bakker, and Dylan Molenaar. Willingness to share research data is related to the strength of the evidence and the quality of reporting of statistical results. PLoS One, 6(11):e26828, November 2011.
- F55
Mark D Wilkinson, Michel Dumontier, I Jsbrand Jan Aalbersberg, Gabrielle Appleton, Myles Axton, Arie Baak, Niklas Blomberg, Jan-Willem Boiten, Luiz Bonino da Silva Santos, Philip E Bourne, Jildau Bouwman, Anthony J Brookes, Tim Clark, Mercè Crosas, Ingrid Dillo, Olivier Dumon, Scott Edmunds, Chris T Evelo, Richard Finkers, Alejandra Gonzalez-Beltran, Alasdair J G Gray, Paul Groth, Carole Goble, Jeffrey S Grethe, Jaap Heringa, Peter A C 't Hoen, Rob Hooft, Tobias Kuhn, Ruben Kok, Joost Kok, Scott J Lusher, Maryann E Martone, Albert Mons, Abel L Packer, Bengt Persson, Philippe Rocca-Serra, Marco Roos, Rene van Schaik, Susanna-Assunta Sansone, Erik Schultes, Thierry Sengstag, Ted Slater, George Strawn, Morris A Swertz, Mark Thompson, Johan van der Lei, Erik van Mulligen, Jan Velterop, Andra Waagmeester, Peter Wittenburg, Katherine Wolstencroft, Jun Zhao, and Barend Mons. The FAIR guiding principles for scientific data management and stewardship. Scientific Data, 3:160018, March 2016.
- F56
Greg Wilson, Jennifer Bryan, Karen Cranston, Justin Kitzes, Lex Nederbragt, and Tracy K Teal. Good enough practices in scientific computing. PLoS Computational Biology, 13(6):e1005510, June 2017.
- F57
The Turing Way Community, Becky Arnold, Louise Bowler, Sarah Gibson, Patricia Herterich, Rosie Higman, Anna Krystalli, Alexander Morley, Martin O'Reilly, and Kirstie Whitaker. The turing way: a handbook for reproducible data science. 2019.
- 1
Sources: Icons from the Noun Project: Data Sharing by Design Circle; Share Code by Danil Polshin; Data by Nirbhay; Publication by Adrien Coquet; Broadcast by Amy Chiang; Logos: used with permission by the copyright holders.