Study inception, planning, and ethics
Study inception, planning, and ethics#
Issue
Each individual decision from the beginning of the study will contribute to facilitate or hamper reproducibility.
What do we provide
In the current section, we will describe practices and tools for preparation, piloting, pre-registration, obtaining participants’ consent and ongoing quality control and assessment.
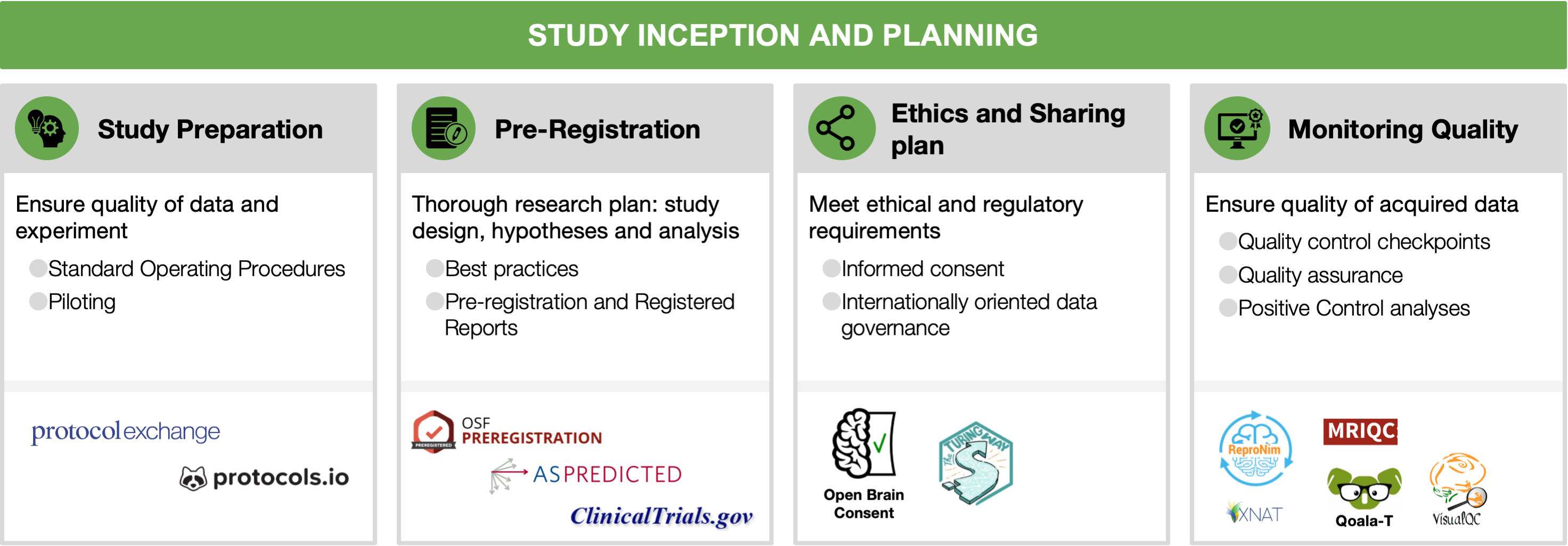
2.1 Study preparation and piloting
Research projects usually begin with descriptions of general, theoretical questions in documents such as grants or thesis proposals. Such foundations are essential but necessarily broad. When the project moves from proposal to implementation, these descriptions are translated into concrete protocols and stimuli, a process that can be streamlined by the incorporation of open procedures and comprehensive piloting. The promise is that the more preparation and piloting is conducted prior to data collection, the more likely it is that the project will be successful: that analyses of its data can contribute to answering its motivating ideas and questions [Strand, 2021].
Standard Operating Procedures (SOPs) can take different forms, and are powerful tools for planning, conducting, recording, and sharing projects. Ideally, SOPs describe the entire data collection procedure (e.g., recruitment, performing the experiment, data storage, preprocessing, quality control analyses), in sufficient detail for a reader to conduct the experiment themselves with minimal supervision, thereby contributing to reproducibility. SOPs may begin as an outline with vague descriptions, preferably during the pilot stage, and then become more detailed over time. For example, if a session is lost due to a button box signal failure, an image of its correct settings could be added to the SOPs. At the end of the project, its SOPs should be released along with its publications and datasets, to provide a source of answers for the detailed procedural information that may be needed for experiment replication or dataset reuse, but are not included in typical publications.
Many resources can assist with experiment planning and SOP creation. Documents and experiences from similar studies conducted locally are valuable, but should not be the only source of information during planning, since, for example, a procedure may be considered standard in one institution but not in another. Public SOPs can serve as examples, as can protocols published on specialized sites (e.g., Protocol Exchange, protocols.io, Nature Protocols; see Table S1). Best practices guides are now available for many imaging modalities (MRI: [Nichols et al., 2017]; MEG/EEG: [Pernet et al., 2020]; fNIRS: [Yücel et al., 2021]; PET: [Knudsen et al., 2020]). Open resources for stimulus presentations and behavioral data acquisition are also recommended to increase reproducibility (see Section 3.2).
Piloting should be considered an integral part of the planning process. By “piloting” we mean the acquisition and evaluation of data prior to the collection of the actual experimental data; verifying the feasibility of the whole research workflow. While it is not a necessary prerequisite for reproducibility, it is a good scientific practice to produce higher quality research, and facilitates reproducibility via better documentation and SOPs. A piloting stage before starting data collection is important, not only for ensuring that the protocol will go smoothly when the first participant arrives, but also that the SOPs are complete and, critically, that the planned analyses can be carried out with the actual experimental data recorded. For example, pilot tests may be set up to confirm that the task produces the expected behavioral patterns (e.g., faster reaction time in condition A than B), that the log files are complete, and that image acquisition can be synchronized with stimulus presentation. Piloting should also include testing the data storage and retrieval strategies, which may include storing consent documents (see Section 2.3), questionnaire responses, imaging data, and quality control reports. SOPs also prescribe how data will be organized, preferably according to a schema (e.g., the Brain Imaging Data Structure, see Section 4.1). Data organization largely determines the efficient implementation of analysis pipelines and improved reproducibility, reusability, and shareability of the data and results.
Analyses of the pilot data are very important and can take several forms. One is to test for the effects of interest: establishing that the desired analyses can be performed and that the data quality and effect sizes are sufficient to produce valid and reproducible results (see Section 2.2 and see 2.4; for power estimation tools see the resources table). A second type of pilot analysis is to establish tests for effects not of direct interest, but suitable for controls. As discussed further in section 2.4, positive control analyses involve strong, well-understood effects that must be present in a valid dataset. It is worth mentioning that well structured and documented openly available datasets (see Section 4) could also serve for analysis piloting though they would lack the test for potential site specific technical issues. Simulations could also be used to ensure that the planned analysis is doable and valid.
2.2 Preregistration
Pre-registration is the specification of the research plan in advance, prior to data collection or at least prior to data analysis [Nosek and Stephen Lindsay, 2018]. Pre-registration usually includes the study design, the hypotheses and the analysis plan. It is submitted to a registry, resulting in a frozen time-stamped version of the research plan. Its main aim is to distinguish between hypothesis-testing (confirmatory) research and hypothesis-generating (exploratory) research. While both are necessary for scientific progress, they require different tests and the conclusions that can be inferred based on them are different [Nosek et al., 2018].
Registered reports is a relatively novel publishing format that can be seen as advanced pre-registration. This format is becoming very common, with a growing number of hundreds of participating journals [Chambers, 2019, Hardwicke and Ioannidis, 2018]. In a registered report, a detailed pre-registration is submitted to a specific journal, including introduction, planned methods and potentially preliminary data. Then, it goes through peer review prior to data collection (or prior to data analysis in certain cases, for example for studies that rely on large-scale publicly shared data). If the proposed plan is approved following peer review, it receives an “in-principle acceptance”, indicating that if the researchers follow their accepted plan, and their conclusions fit their findings, their paper will be published. An in-principle accepted registered report is sometimes required to be additionally pre-registered. Recently, a platform for peer review of registered reports preprints was launched, named “Peer Community in registered reports” (see the resources table).
There are many benefits to pre-registration, from the field to the individual level. Transparency with regard to the research plan, and whether an analysis is confirmatory or exploratory, increases the credibility of scientific findings. It helps to mitigate some of the effects of human biases on the scientific process, and reduces analytical flexibility, p-hacking [Simmons et al., 2011] and hypothesizing after the results are known [Munafò et al., 2017, Nosek et al., 2019, Kerr, 1998, Nature, 2015]. There is initial evidence that the quality of pre-registered research is judged higher than in conventional publications [Soderberg et al., 2021]. Nonetheless, it should be noted that pre-registration is not sufficient to fully protect against questionable research practices [Devezer et al., 2021, Paul et al., 2021, Rubin, 2020] and their general impact will depend on the extent journals will implement them. Registered reports also mitigate publication bias by accepting papers based on hypothesis and methods, independently of the findings, and indeed it has been shown that pre-registration and registered reports lead to more published null findings [Allen and Mehler, 2019, Kaplan and Irvin, 2015, Scheel, 2020] and report lower effect sizes [Allen and Mehler, 2019, Kaplan and Irvin, 2015, Scheel, 2020]. For the individual researcher, registered reports with a two-stage review are an excellent example in which authors benefit from feedback on their methods before even starting data collection. They can help improve the research plan and spot mistakes in time, and provide assurance that the study will be published [Kidwell et al., 2016, Wagenmakers and Dutilh, 2016]. It should be noted, though, that registered reports require a significant time commitment, that is likely to pay off in the long-term but is not easily accommodated in many traditional project funding models.
While pre-registration is not the common practice yet, it is becoming more common over time [Nosek and Stephen Lindsay, 2018] and requirements by journals and funding agencies are already changing. There are many available templates and forms for pre-registration, organized by discipline or study types, for example for fMRI and EEG (see the resources table), and published guidelines for pre-registration in EEG are also available [Paul et al., 2021]. There are different approaches with respect to what should be pre-registered. For instance, some believe it should be an exhaustive description of the study, including the background and justification for the research plan, while others believe it should be short and concise, including only the necessary details to reduce the likelihood of p-hacking and allowing reviewers to review it properly during the peer review process [Simmons et al., 2021]. Pre-registration could also be more flexible and adaptive by pre-registering contingency plans or complex decision trees [Benning et al., 2019].
Once researchers develop an idea and design a study, they can write and pre-register their research plan [Nosek and Stephen Lindsay, 2018, Paul et al., 2021]. Pre-registration could be performed following the piloting stage (Section 2.1), but studies can be pre-registered irrespective of whether they include a piloting stage or not. There are many online registries where researchers can pre-register their study. The three most frequently used platforms are: (1) OSF, a platform that can also be used to share additional information about the study/project (such as data and code), with multiple templates and forms for different types of pre-registration, in addition to extensive resources about pre-registration and other open science practices; (2) aspredicted.org, a simplified form for pre-registration (Simmons, Nelson, and Simonsohn 2021); and (3) clinicaltrials.gov, which is used for registration of clinical trials in the U.S. (see the resources table).
Once the pre-registration is submitted, it can remain private or become public immediately, depending on the platform and the researcher’s preferences. Then, the researcher collects the data and executes the research plan. When writing the manuscript to report the study, the researcher is advised to include a link to the pre-registration, clearly and transparently describe and justify any deviation from the pre-registered plan and also report all registered analyses. Additional analyses to deepen some results or look into unexpected effects are encouraged, and are part of the normal scientific investigation. The added benefit of pre-registration is that such analyses do not need to reach pre-specified levels of significance because they are reported as exploratory.
2.3 Ethical review and data sharing plan
The optimism of the scientific community about improving science by making all research assets open and transparent has to take into account privacy, ethics and the associated legal and regulatory needs for each institution and country. Whereas on the one hand sharing data (most often collected with public funds) is critical to advance science, on the other hand, sharing data can in some situations become infeasible to safeguard privacy. Data governance concerns the regulatory and ethical aspects of data management and sharing of data files, metadata and data-processing software (see sections 6.1, 6.2 and 6.3). When data sharing crosses national borders, data governance is called International Data Governance (IDG). IDG depends on ethical, cultural and international laws.
Data sharing is beneficial for both reproducibility and the exploration and formulation of new hypotheses. Therefore, it is important to ensure, prior to data collection, that the collected data could be later shared. Consent forms for neuroimaging studies should contain, or be accompanied with, a specific section to allow data sharing. Such data sharing forms should include information about where and in what form the data will be shared, as well as who will have access and how restrictive this access will be. Data sharing forms should also make explicit how these factors determine to what extent a later withdrawal or editing of the data on the repository is possible. Additional requirements might be in place depending on the location where the research is being conducted. Given the international nature of the majority of neuroscience projects, IDG has become a priority [Eiss, 2020]. Further work will be needed to implement an IDG approach that can facilitate research while protecting privacy and ethics. Specific recommendations on how to implement IDG have been proposed by [Eke et al., 2022].
Open and reproducible neuroimaging thus starts by (1) planning which data would be collected; (2) planning how these data would later be shared; (3) having ethical and legal clearance to share data; but also (4) the infrastructural means for this sharing (for more information about data sharing and available platforms, see Section 6; for data governance see Section 6). The informed consent should include a statement about the research goals, inform about the experimental procedures, its expected duration, potential risks, who can participate in a study, and potential conflicts of interest if they exist. It should also inform participants that participation is voluntary, and that the consent can be withdrawn at any time during the experiment without a disadvantage for the participant. If limited intervals for consent withdrawal exist, they should be clearly stated. Furthermore, it should also include a statement that the participant understood the information provided and agreed to participate. Clinical research may require adherence to additional, country specific regulations.
Since 2014 the Open Brain Consent project (see the resources table), which was founded under the ReproNim project umbrella, provides examples and templates translated to multiple languages to help researchers prepare consent forms, including the recent development of an EU GDPR-compliant template (The Open Brain Consent working group 2021). When planning the recruitment procedures, it is important to aim for equity, diversity and inclusivity [Forbes et al., 2021, Henrich et al., 2010], avoiding obtaining results that may not generalize to larger populations and improving the quality of research (e.g., [Baggio et al., 2013]).
2.4 Looking at the data early and often: monitoring quality
Inevitably, unexpected events and errors will occur during every experiment and in every part of the research workflow. These can take many forms, including dozing participants, hardware malfunction, data transfer errors, and mislabeled results files. As data progresses through the workflow, issues are likely to cascade and amplify, perhaps masking or mediating experimental effects, thereby damaging the reliability of the results. The impact of such surprises can range from the trivial and easily corrected to the catastrophic, rendering the collected data unusable or conclusions drawn from it invalid. Identifying issues and errors as early as possible is important to enable adding corrective measures to the protocol, but also because some issues are much easier to detect when the data are in a less-processed form. For example, a number of typical artifacts in anatomical MRI are known to be easier to identify in the background of the image and regions of no-interest [Mortamet et al., 2009], and can easily remain undetected if the first quality control check is set up after, e.g., brain extraction, which masks out non brain tissue. Thus, it is fundamental to pre-establish within the SOPs (section 2.1) the mechanisms set in place to ensure the quality of the study. There are several mechanisms available that help to ensure that all required data are being recorded with sufficient quality and in a way that makes them analyzable.
Quality control checkpoints. Establishing quality control (QC) checkpoints [Strother, 2006] is necessary for every project: which data are usable for analyses, and which are not? At these key points in the preprocessing or analysis workflow the data’s quality is checked, and if insufficient, it does not move on to the next stage. Low quality data are much less likely to be reproducible with new data or methods. Critically, the exclusion criteria of each checkpoint must be defined in advance (preferably stated in the SOPs and the pre-registration document, see sections 2.1 and 2.2) to preempt unintentional cherry-picking (i.e., excluding data points to reinforce the results), which is a major contributor to irreproducibility via undisclosed flexibility. Some criteria are widely accepted and applicable, for example, that all neuroimaging data should be screened to eliminate clear artifacts, such as data corrupted by incidental electromagnetic interference or participants movements. A similarly well-established checkpoint of the workflow is visualizing and inspecting the outputs of surface reconstruction methods in MRI, checking time activity curves in high binding regions for PET or power spectral content in MEG and EEG; these fundamental QC checkpoints and their implementation are heavily dependent on the immediately previous processing step. Such QC may be conducted manually by experts using software aids, like visual summary reports or visualization software such as MRIQC [Esteban et al., 2017]. More objective, automatic exclusion criteria, are currently an open and active line of work in neuroimaging (e.g., [Ding et al., 2019, Esteban et al., 2019, Kollada et al., 2021]). Some QC checkpoints, such as for acceptable task performance or participant movement, are often defined for individual tasks, experiments and hypotheses.
Quality assurance (QA). Tracking QC decisions will also enable identifying structured failures and artifacts that require not just excluding affected datasets, but rather taking corrective actions to preempt propagation to additional datasets. When a mishap occurs, the experimenters should investigate its cause, and if possible, change the SOPs (Section 2.1) and related materials to reduce the chance of it happening again. For example, if many participants report confusion about task instructions, the training procedure and experimenter script could be altered. Automated checks and reports can be very effective, such as real-time monitoring of participant motion during data collection [Heunis et al., 2020], or validating that image parameters are as expected before storage (e.g., with XNAT [Marcus et al., 2007] or ReproNim tools [Kennedy et al., 2019]).
Positive control analyses. A final aspect of quality assurance is the incorporation of positive control analyses: analyses included not because they are of interest for the scientific questions, but because they provide evidence that the dataset is of sufficient quality to conduct the analyses of interest, and that the analysis is valid. Ideally, positive control analyses focus on strong, well-established effects that must be present if the dataset is valid. For example, with task fMRI designs, button pressing, which should be associated with contralateral motor activation, is often a convenient target for positive control analysis. In MEG and EEG, participants can be asked to blink their eyes, open their mouths, or clench their jaws, and the recordings checked for the associated artifacts. Positive control analyses should also be carried out during piloting, when changes to the protocol are still possible (see Section 2.1). For example, if button presses are not clearly detectable during piloting, the acquisition sequence may not have sufficient SNR for the planned analyses and so need modification. Positive controls can further serve for analysis pipeline optimization prior to conducting the optimized analysis on the outcome of interest, thus preventing legitimate optimization from turning into p-hacking.
References on this page
- B1(1,2)
Christopher Allen and David M A Mehler. Open science challenges, benefits and tips in early career and beyond. PLoS Biology, 17(5):e3000246, May 2019.
- B2
Giovannella Baggio, Alberto Corsini, Annarosa Floreani, Sandro Giannini, and Vittorina Zagonel. Gender medicine: a task for the third millennium. Clinical Chemistry and Laboratory Medicine, 51(4):713–727, April 2013.
- B3
Stephen D Benning, Rachel L Bachrach, Edward A Smith, Andrew J Freeman, and Aidan G C Wright. The registration continuum in clinical science: a guide toward transparent practices. J. Abnorm. Psychol., 128(6):528–540, August 2019.
- B4
Chris Chambers. What's next for registered reports? Nature, 573(7773):187–189, September 2019.
- B5
Berna Devezer, Danielle J Navarro, Joachim Vandekerckhove, and Erkan Ozge Buzbas. The case for formal methodology in scientific reform. Royal Society Open Science, 8(3):200805, March 2021.
- B6
Yang Ding, Sabrina Suffren, Pierre Bellec, and Gregory A Lodygensky. Supervised machine learning quality control for magnetic resonance artifacts in neonatal data sets. Hum. Brain Mapp., 40(4):1290–1297, March 2019.
- B7
Robert Eiss. Confusion over europe's data-protection law is stalling scientific progress. Nature, 584(7822):498, August 2020.
- B8
Damian Eke, Amy Bernard, Jan G Bjaalie, Ricardo Chavarriaga, Takashi Hanakawa, Anthony Hannan, Sean Hill, Maryann Elizabeth Martone, Agnes McMahon, Oliver Ruebel, Edda Thiels, and Franco Pestilli. International data governance for neuroscience. Neuron, 110(4):600–612, 2022.
- B9
Oscar Esteban, Daniel Birman, Marie Schaer, Oluwasanmi O Koyejo, Russell A Poldrack, and Krzysztof J Gorgolewski. MRIQC: advancing the automatic prediction of image quality in MRI from unseen sites. PLoS One, 12(9):e0184661, September 2017.
- B10
Oscar Esteban, Ross W Blair, Dylan M Nielson, Jan C Varada, Sean Marrett, Adam G Thomas, Russell A Poldrack, and Krzysztof J Gorgolewski. Crowdsourced MRI quality metrics and expert quality annotations for training of humans and machines. Sci Data, 6(1):30, April 2019.
- B11
Samuel H Forbes, Prerna Aneja, and Olivia Guest. The myth of (a)typical development. PsyArXiv, October 2021.
- B12
Tom E Hardwicke and John P A Ioannidis. Mapping the universe of registered reports. Nature Human Behaviour, 2(11):793–796, November 2018.
- B13
Joseph Henrich, Steven J Heine, and Ara Norenzayan. The weirdest people in the world? Behavioral and Brain Sciences, 33(2-3):61–83, June 2010.
- B14
Stephan Heunis, Rolf Lamerichs, Svitlana Zinger, Cesar Caballero-Gaudes, Jacobus F A Jansen, Bert Aldenkamp, and Marcel Breeuwer. Quality and denoising in real-time functional magnetic resonance imaging neurofeedback: a methods review. Human Brain Mapping, 41(12):3439–3467, August 2020.
- B15(1,2)
Robert M Kaplan and Veronica L Irvin. Likelihood of null effects of large NHLBI clinical trials has increased over time. PLoS One, 10(8):e0132382, August 2015.
- B16
David N Kennedy, Sanu A Abraham, Julianna F Bates, Albert Crowley, Satrajit Ghosh, Tom Gillespie, Mathias Goncalves, Jeffrey S Grethe, Yaroslav O Halchenko, Michael Hanke, Christian Haselgrove, Steven M Hodge, Dorota Jarecka, Jakub Kaczmarzyk, David B Keator, Kyle Meyer, Maryann E Martone, Smruti Padhy, Jean-Baptiste Poline, Nina Preuss, Troy Sincomb, and Matt Travers. Everything matters: the ReproNim perspective on reproducible neuroimaging. Front. Neuroinform., 13:1, February 2019.
- B17
N L Kerr. HARKing: hypothesizing after the results are known. Personality and Social Psychology Review, 2(3):196–217, 1998.
- B18
Mallory C Kidwell, Ljiljana B Lazarević, Erica Baranski, Tom E Hardwicke, Sarah Piechowski, Lina-Sophia Falkenberg, Curtis Kennett, Agnieszka Slowik, Carina Sonnleitner, Chelsey Hess-Holden, Timothy M Errington, Susann Fiedler, and Brian A Nosek. Badges to acknowledge open practices: a simple, low-cost, effective method for increasing transparency. PLoS Biology, 14(5):e1002456, May 2016.
- B19
Gitte M Knudsen, Melanie Ganz, Stefan Appelhoff, Ronald Boellaard, Guy Bormans, Richard E Carson, Ciprian Catana, Doris Doudet, Antony D Gee, Douglas N Greve, Roger N Gunn, Christer Halldin, Peter Herscovitch, Henry Huang, Sune H Keller, Adriaan A Lammertsma, Rupert Lanzenberger, Jeih-San Liow, Talakad G Lohith, Mark Lubberink, Chul H Lyoo, J John Mann, Granville J Matheson, Thomas E Nichols, Martin Nørgaard, Todd Ogden, Ramin Parsey, Victor W Pike, Julie Price, Gaia Rizzo, Pedro Rosa-Neto, Martin Schain, Peter Jh Scott, Graham Searle, Mark Slifstein, Tetsuya Suhara, Peter S Talbot, Adam Thomas, Mattia Veronese, Dean F Wong, Maqsood Yaqub, Francesca Zanderigo, Sami Zoghbi, and Robert B Innis. Guidelines for the content and format of PET brain data in publications and archives: a consensus paper. Journal of Cerebral Blood Flow and Metabolism, 40(8):1576–1585, August 2020.
- B20
Matthew Kollada, Qingzhu Gao, Monika S Mellem, Tathagata Banerjee, and William J Martin. A generalizable method for automated quality control of functional neuroimaging datasets. In Arash Shaban-Nejad, Martin Michalowski, and David L Buckeridge, editors, Explainable AI in Healthcare and Medicine: Building a Culture of Transparency and Accountability, pages 55–68. Springer International Publishing, Cham, 2021.
- B21
Daniel S Marcus, Timothy R Olsen, Mohana Ramaratnam, and Randy L Buckner. The extensible neuroimaging archive toolkit: an informatics platform for managing, exploring, and sharing neuroimaging data. Neuroinformatics, 5(1):11–34, 2007.
- B22
Bénédicte Mortamet, Matt A Bernstein, Clifford R Jack, Jr, Jeffrey L Gunter, Chadwick Ward, Paula J Britson, Reto Meuli, Jean-Philippe Thiran, Gunnar Krueger, and Alzheimer's Disease Neuroimaging Initiative. Automatic quality assessment in structural brain magnetic resonance imaging. Magn. Reson. Med., 62(2):365–372, August 2009.
- B23
Marcus R Munafò, Brian A Nosek, Dorothy V M Bishop, Katherine S Button, Christopher D Chambers, Nathalie Percie Du Sert, Uri Simonsohn, Eric Jan Wagenmakers, Jennifer J Ware, and John P A Ioannidis. A manifesto for reproducible science. Nature Human Behaviour, 1(1):1–9, 2017.
- B24
Thomas E Nichols, Samir Das, Simon B Eickhoff, Alan C Evans, Tristan Glatard, Michael Hanke, Nikolaus Kriegeskorte, Michael P Milham, Russell A Poldrack, Jean-Baptiste Poline, Erika Proal, Bertrand Thirion, David C Van Essen, Tonya White, and B T Thomas Yeo. Best practices in data analysis and sharing in neuroimaging using MRI. Nature Neuroscience, 20(3):299–303, February 2017.
- B25
Brian A Nosek, Emorie D Beck, Lorne Campbell, Jessica K Flake, Tom E Hardwicke, David T Mellor, Anna E van 't Veer, and Simine Vazire. Preregistration is hard, and worthwhile. Trends in Cognitive Sciences, 23(10):815–818, October 2019.
- B26
Brian A Nosek, Charles R Ebersole, Alexander C DeHaven, and David T Mellor. The preregistration revolution. Proceedings of the National Academy of Sciences, 2017(15):201708274, 2018.
- B27(1,2,3)
Brian A Nosek and D Stephen Lindsay. Preregistration becoming the norm in psychological science. APS Observer, February 2018.
- B28(1,2,3)
Mariella Paul, Gisela Govaart, and Antonio Schettino. Making ERP research more transparent: guidelines for preregistration. International Journal of Psychophysiology, 164:52–63, 2021.
- B29
Cyril R Pernet, Marta I Garrido, Alexandre Gramfort, Natasha Maurits, Christoph M Michel, Elizabeth Pang, Riitta Salmelin, Jan Mathijs Schoffelen, Pedro A Valdes-Sosa, and Aina Puce. Issues and recommendations from the OHBM COBIDAS MEEG committee for reproducible EEG and MEG research. Nature Neuroscience, 23(12):1473–1483, December 2020.
- B30
Mark Rubin. Does preregistration improve the credibility of research findings? The Quantitative Methods in Psychology, 16(4):376–390, 2020.
- B31(1,2)
Anne M Scheel. Registered reports: a process to safeguard high-quality evidence. Quality of Life Research, 29(12):3181–3182, December 2020.
- B32
Joseph P Simmons, Leif Nelson, and Uri Simonsohn. Pre‐registration: why and how. Journal of Consumer Psychology, 31(1):151–162, January 2021.
- B33
Joseph P Simmons, Leif D Nelson, and Uri Simonsohn. False-positive psychology: undisclosed flexibility in data collection and analysis allows presenting anything as significant. Psychological Science, 22(11):1359–1366, 2011.
- B34
Courtney K Soderberg, Timothy M Errington, Sarah R Schiavone, Julia Bottesini, Felix Singleton Thorn, Simine Vazire, Kevin M Esterling, and Brian A Nosek. Initial evidence of research quality of registered reports compared with the standard publishing model. Nature Human Behaviour, 5(8):990–997, August 2021.
- B35
Julia Strand. Error tight: exercises for lab groups to prevent research mistakes. PsyArXiv, 2021.
- B36
Stephen C Strother. Evaluating fMRI preprocessing pipelines. IEEE Engineering in Medicine and Biology, 25(2):27–41, March 2006.
- B37
Eric-Jan Wagenmakers and Gilles Dutilh. Seven selfish reasons for preregistration. APS Observer, October 2016.
- B38
Meryem A Yücel, Alexander V Lühmann, Felix Scholkmann, Judit Gervain, Ippeita Dan, Hasan Ayaz, David Boas, Robert J Cooper, Joseph Culver, Clare E Elwell, Adam Eggebrecht, Maria A Franceschini, Christophe Grova, Fumitaka Homae, Frédéric Lesage, Hellmuth Obrig, Ilias Tachtsidis, Sungho Tak, Yunjie Tong, Alessandro Torricelli, Heidrun Wabnitz, and Martin Wolf. Best practices for fNIRS publications. Neurophotonics, 8(1):012101, January 2021.
- B39
Nature. Let's think about cognitive bias. Nature, 526(7572):163, October 2015.
- 1
Sources: Icons from the Noun Project: Registration by WEBTECHOPS LLP; Shared by arjuazka; Computer warranty by Thuy Nguyen; Logos: used with permission by the respective copyright holders.